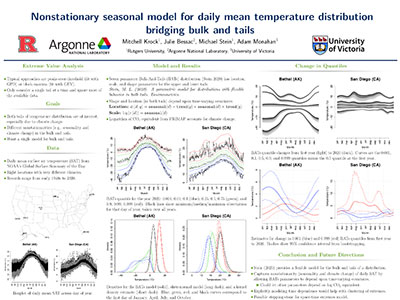
Non-stationary Seasonal Model for Daily Mean Temperature Distribution Bridging Bulk and Tails
Mitchell Krock, Postdoctoral Student
Rutgers University
Abstract
In traditional extreme value analysis, the bulk of the data is ignored, and only the tails of the distribution are used for inference. Extreme observations are specified as values that exceed a threshold or as maximum values over distinct blocks of time, and subsequent estimation procedures are motivated by asymptotic theory for extremes of random processes.
For environmental data, non-stationary behavior in the bulk of the distribution, such as seasonality or climate change, will also be observed in the tails. To accurately model such non-stationarity, it seems natural to use the entire dataset rather than just the most extreme values. It is also common to observe different types of non-stationarity in each tail of a distribution. Most work on extremes only focuses on one tail of a distribution, but for temperature, both tails are of interest. This paper builds on a recently proposed parametric model for the entire probability distribution that has flexible behavior in both tails. We apply an extension of this model to historical records of daily mean temperature at several locations across the United States with different climates and local conditions. We highlight the ability of the method to quantify changes in the bulk and tails across the year over the past decades and under different geographic and climatic conditions.
The proposed model shows good performance when compared to several benchmark models that are typically used in extreme value analysis of temperature.